New Brain-Like Computing Device Simulates Human Learning
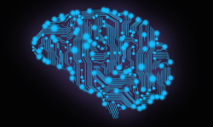
Image Courtesy of Health Transformer.com
May 5, 2021
Researchers from Northwestern University and the University of Hong Kong developed a new device containing “synaptic transistors”, which are small devices that process and store data in a way that mimics the human brain. After connecting transistors into a device, researchers conditioned it to associate light with pressure — similar to how Pavlov’s dog associated a bell with food.
With this cutting-edge engineering, synaptic transistors surpass the limitations of traditional computing.
The researchers demonstrated that the transistor could mimic both the short-term and long-term plasticity of synapses in the human brain, building on memories to instill over time. In the synaptic transistor, ions behave similarly to neurotransmitters, sending signals between terminals to form an artificial synapse. By retaining data from the trapped ions, the transistor remembers previous activities, developing long-term plasticity, thus forming its own memories.
The researchers demonstrated their device’s synaptic behavior by connecting single synaptic transistors into a circuit to simulate associative learning. They inputted pressure and light sensors into the circuit and trained the circuit to associate the two sensors with one another.
After one training cycle, the circuit made an initial connection between light and pressure. After five training cycles, the circuit significantly associated light with pressure. Light, alone, was able to trigger a signal.
Because the synaptic circuit contains similar material to that of plastic, it can be readily fabricated and easily integrated into soft, wearable electronics, AI robotics, and implantable devices for direct, physical contact with living tissue.
This device will enhance the next generation of bioelectronics, thanks to Northwestern’s Jonathan Rivnay, a senior author of the study, Paddy Chan, an associate professor of mechanical engineering at the University of Hong Kong, and Xudong Ji, a postdoctoral researcher in Rivnay’s group.